The Path to Data Centricity 4: Why a Data Hub
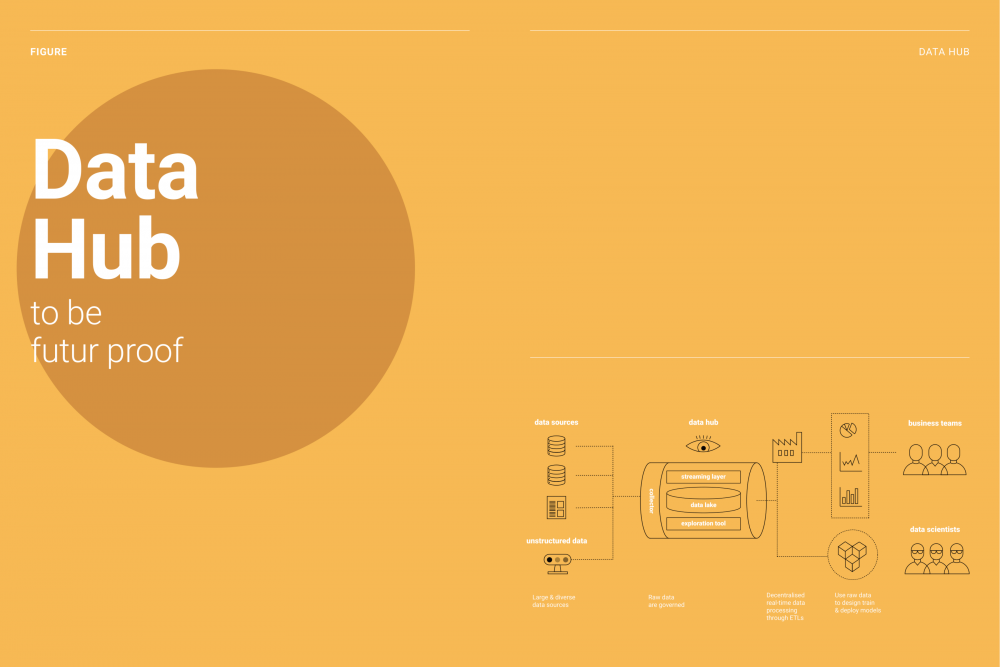
You want to predict the churn and to take action in real time to retain customers who are showing signs of dropping out. You have to feed your data warehouse with raw and transformed data, often in real time, feeding machine learning models that are directly embedded in applications.
The data lake has become a liability as it struggles to keep up with the load of data it has to store while supporting the reliability constraint of the prediction model.
Data hubs are a strategic intermediary between producers and consumers of data. While data lakes take care of the collection and storing of data, they can’t keep up with the processing capabilities required for the model execution. We advise to dissociate the storing from the distribution of data, as this increases the scalability. In this hub configuration, data lakes can become places that merely store and index data to make them available for exploration.
The advantages of a data-hub architecture are:
- All data lake and data warehouse advantages
- Real-time data management: streaming and the feeding of data to applications in real time
- Managed data lake boosted by a streaming platform
- Integrates well with a pre-existing data warehouse
However, keep in mind that:
- The data hub pattern does not describe how to govern data; it eases data governance but does not provide it.
- Streaming is a relatively young technology.
- Splitting the storing from the distribution means that you need to find a way to distribute data without creating a bottleneck in the data lake during the distribution stage.
Our Recommendation:
Data hubs should be the go-to architecture for any company looking at expanding the use of data outside their existing data warehouse. If you want to power a digital platform with data, to use machine learning, to guarantee integrity and consistency between data duplicates, to understand your customer better, and to provide context-aware services, the data hub architecture is the way to go.
Data hubs can be implemented in many ways. We can help you choose the best type of data hub architecture for your specific data sharing use cases by focusing on the key characteristics of each hub type category.
We have defined our own data architecture vision based on the data hub concept for our clients to collect data from multiple sources, then distribute it to applications and users for all their possible needs. Our data architecture experts have built data hubs for different industries, and our data engineers have implemented many instances of that data architecture vision.
To make it possible for our clients to save time and costs on their journey towards data centricity, we designed an off-the-shelf data hub.
Coming up: The Path to Data Centricity 5: Why a Data Mesh
This article is the first in a series of blog posts in which we highlight the differences between storing solutions to support your data-driven strategy. In the next part, we will discuss (governed) data meshes.
You can also read our white paper that walks you through the different solutions available and help you make a choice based on your business needs.