The Drivers of AI Success in 2021
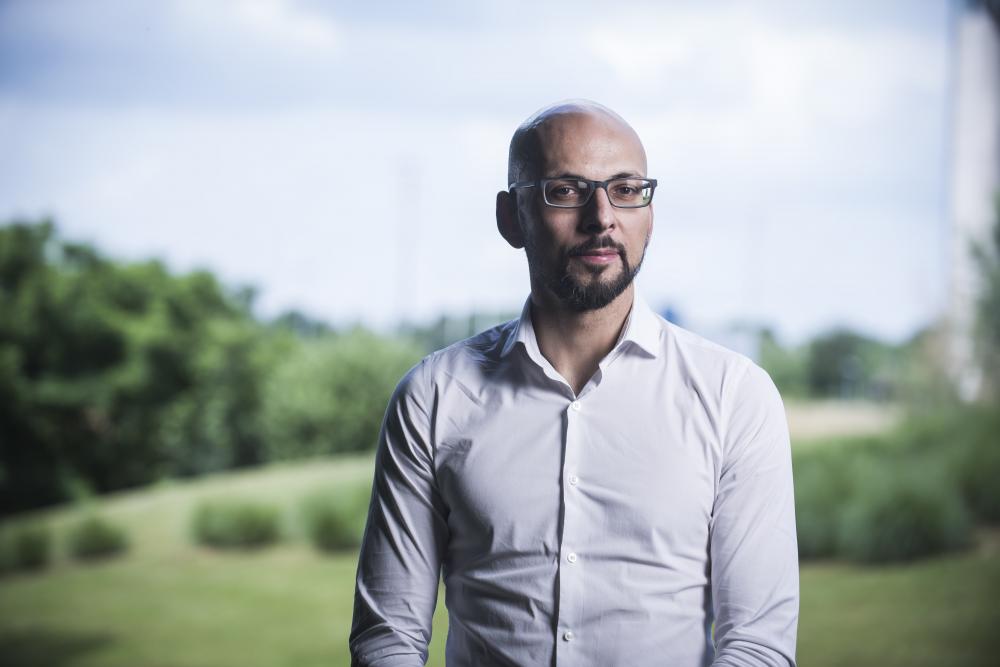
Our research director Sabri Skhiri comes back on the three practices in which you should focus time to capitalize on AI technology and generate value.
Leadership and organisation
They are essential to achieve success. You have to choose which factor is critical to your digital transformation project, and where to focus the first efforts, capabilities and resources. Data maturity models are tools that help us translate your goals into an adequate technical target state. Like a compass, they help companies navigate during their digital transformation.
Standard process and foundational components
Going from data exploration to running in production in a smooth and optimised way remains a challenge. It requires a robust data strategy and integrates a wide range of technologies to reach a single point of truth. Streaming data platforms help extract value from data at an affordable cost for the organisation. Finally, although most of the principles and practices of continuous software deployment can be applied to machine learning, AI deployment in production implies unique challenges that need to be handled differently. These specific practices are called MLOps, and the integration of MLOps practices during the model's strategic deployment is essential to deliver value.
Explainability and regulatory compliance
After cybersecurity, they pose the most critical risk. As expected, privacy by design represents a significant challenge for enterprises. Practically all businesses have privacy policies in place, but ensuring their implementation is often difficult and costly. They need a fully automated self-service data platform where the data access policy management is fully GDPR compliant.
If you are interested in learning more about the drivers of AI success for your business, do not hesitate to reach out.
For more details, read McKinsey report on the state of artificial intelligence in 2020.