The Critical Role of Governance in Ensuring Sustainable Success for AI Adoption
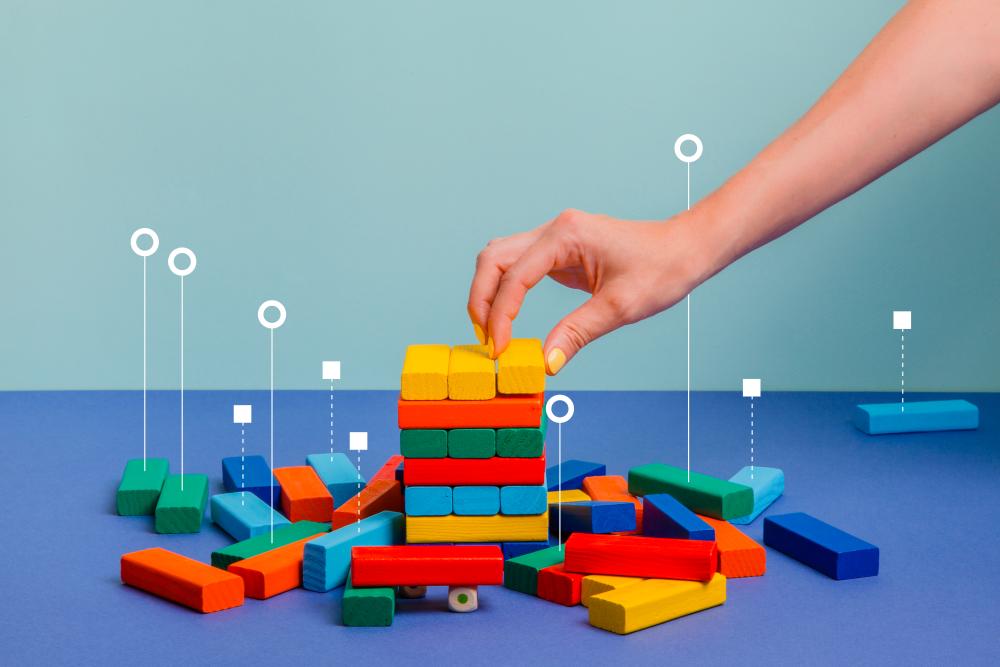
By Carmen Cercelescu
AI adoption is massive today, a trend evident among our clients, including those who are typically more conservative or slow to adopt new technologies. McKinsey's latest AI report confirms our observation, showing a significant jump in adoption, rising to 75% this year from 50% in previous years.
This remarkable growth underscores AI's potential to redefine how businesses operate, offering unprecedented advantages in operational efficiency, decision-making, customer personalisation, and accelerated innovation. Advantages that help our clients stay competitive and rapidly grow in a constantly evolving market.
The Need for AI Governance
However, this rapid embrace of AI technologies comes with its unique set of challenges, particularly in the realm of governance. With AI systems increasingly becoming central to business operations, the absence of an efficient governance framework can lead to substantial risks, including compliance breaches, ethical controversies, and operational discrepancies. Let's dive into a closer examination of the specific governance challenges posed by AI:
1. Systematic Inventory of AI Systems
Many organisations do not have a centralised repository of all their AI tools and applications in use, which complicates the management of updates, version control, and dependencies. Establishing a systematic inventory process is crucial to address this issue. This would involve documenting each AI system, detailing its purpose, data sources, algorithms employed, and performance metrics. Maintaining such an inventory and keeping track of the AI systems in the organisation through regular audits and updates ensures that all AI systems are up-to-date and functioning as intended.
2. Risk Assessment with a Risk-Based Approach
Evaluating AI systems' risks is essential, and it necessitates a nuanced, risk-based approach tailored to the specifics of each application. Rather than relying on simplistic methods such as questionnaires or broad statements of fairness, the focus should be on rigorously profiling each AI model’s behaviour using detailed metrics such as accuracy, fairness, and explainability. These metrics are vital not only for internal assessments, but also for meeting regulatory standards under frameworks like the AI Act. By doing this correctly, we believe you can address nearly 70% of the AI Act requirements.
With these metrics established, the next question arises: how are these measurements recognised from a legal point of view?
3. Navigating Legal and Regulatory Frameworks
Navigating this 'legal jungle'—including regulations like the AI Act, Data Act, and NIS2 Directive—requires a deep understanding of both regulatory requirements and their technical implications.
These regulations necessitate a multidisciplinary approach where legal requirements are translated into actionable technical and operational guidelines to ensure seamless governance and compliance.
Effective risk management therefore involves not only technical diligence, but also legal foresight to ensure compliance and operational safety.
Implementation of Robust Governance Practices
To truly harness AI's potential while mitigating associated risks, organisations need to integrate a minimum, robust, governance throughout the AI lifecycle. This involves:
- Business Understanding and Risk Classification: Begin by thoroughly understanding the business goals and classify AI use cases based on AI Act risk classification criteria to align governance efforts with strategic objectives and legal requirements.
- Data Understanding and Policy Compliance: Identify relevant data sources and ensure compliance with data policies, regulations, and legal requirements, including consent management to mitigate legal risks.
- Data Preparation and Governance: Implement robust data governance practices focusing on data quality, ensuring sufficient quality and fairness checks. Address any biases and maintain records of processing activities (RoPAs).
- Modelling and Security: Design AI models with security measures in mind, including robust defences against potential attack vectors. Ensure sensitivity analysis to safeguard against data breaches and unauthorized access.
- Validation and Robustness Testing: Validate AI models rigorously to ensure robustness across diverse datasets and scenarios. Test for accuracy, reliability, and adherence to regulatory standards.
- Deployment and Oversight: Deploy AI systems with human oversight mechanisms in place to monitor performance, intervene when necessary, and ensure ethical compliance. Encourage agile practices to iterate and innovate while maintaining governance standards.
By integrating these steps into the AI lifecycle, organisations can establish a governance framework that not only facilitates innovation and agility but also safeguards against risks, thereby enabling the creation of sustainable value through AI systems.
As expert consultants in AI, data infrastructure, data governance, and legal compliance with a deep understanding of the evolving AI landscape, Euranova is uniquely positioned to help organisations navigate the complexities of the AI Act and implement robust AI governance frameworks. Should your organisation need guidance to operationalise effective data and AI governance practices, do not hesitate to contact us.