Euranova France is Flying Drones: Find Out Why
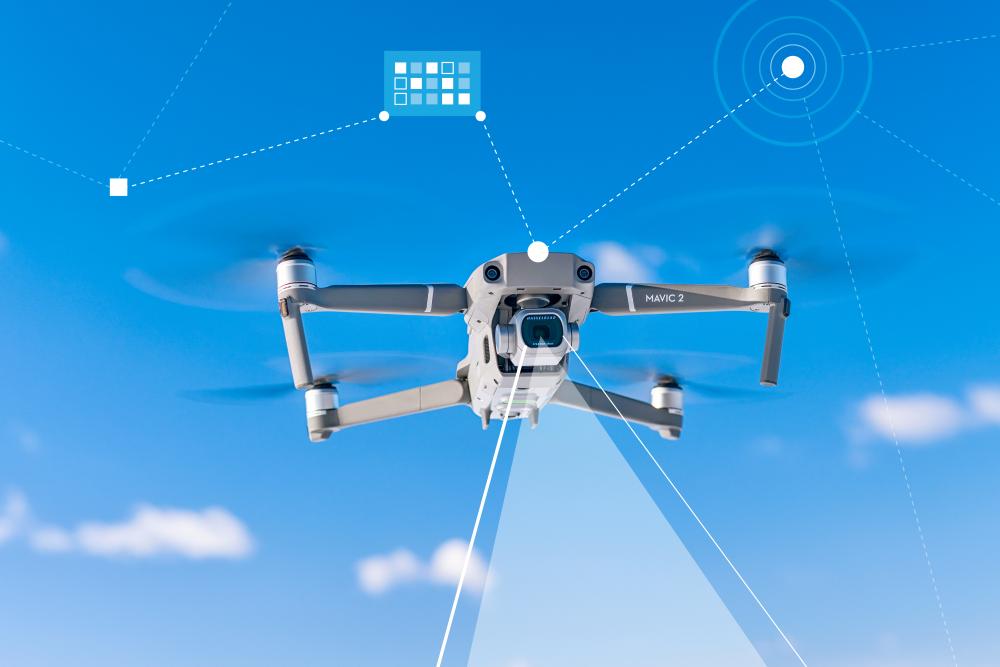
By Quentin Ferré and Guillaume Stempfel
Computer vision can be a game changer for companies in a wide variety of businesses, ranging from start-ups to multinationals, as long as they have access to images and want to extract an understanding of their environment. Now, you would think that this is a common technology for any AI adopters today, but it is not without its challenges.
With the recent progress in machine learning and deep learning, computer vision has boomed. Today, we want to give you some of the best practices in computer vision to learn from to gain new competitive advantages.
So, let's jump in. What is computer vision?
As a quick reminder, computer vision is a field of AI that enables computers and systems to derive meaningful information from digital images, videos and other visual inputs and take actions or make recommendations based on that information. If AI enables computers to think, computer vision enables them to see, observe and understand.
Example applications are vehicle tracking by drones, obstacle detection for autonomous helicopters, medical scanners and X-rays, optimising traffic flows in harbours and airports, 3D environment modelling, tumour detection/evolution prediction, and fake document identification. Since 2018, the team has been reaching the state-of-the-art in this area and has notably been collaborating with clients in the fields of medical imaging, aeronautics and the military.
Challenges in Computer Vision Projects
There are a number of things that companies should know before going for a similar project. Here is one of them: In a data-driven approach, data is key, and the data collection part should not be underestimated! The quality and quantity of this data are crucial to avoid overfitting and build a robust model, and every field study is different. For example, most of the work involving machine learning to model scenes for ground autonomous vehicles requires datasets of videos/images taken from a camera embedded in a car at ground level. That is why an important question we help our clients answer is whether publicly available data sets or the data they already have is sufficient, or if time will have to be allotted to gather new data. Two approaches stand out: the acquisition of real data, for example, via drones, and the creation of synthetic data. It is on this second option, for instance, that we work for a European aircraft manufacturer, providing them with a database of over 200,000 images, including scenes of mountains, deserts or villages, to train their simulation model.
A Case Study
Nexter Robotics, a manufacturer of ground and aero-terrestrial robots, was looking for a way to enable their ground robots to self-locate without GPS, using only their cameras. By cross-referencing the robot's camera view with satellite imagery or drone-acquired aerial photos, the robots could pinpoint their location. Yet, we faced a serious hurdle in developing this computer vision model: data availability. To accumulate the necessary data, we decided to create synthetic data sets focusing on peri-urban environments—a mix of buildings, forests, and fields—tailored to their specific needs. Creating these synthetic scenes was not only cost-effective, but also efficient. In a few days' work, we could generate countless images for training the model. This approach proved practical for training the robots for precise localisation in varied environments, showcasing the cost-effectiveness and practicality of synthetic data in computer vision.
Innovative Solutions for Efficiency and Autonomy
Here is a second challenge you might find most interesting: these systems must be autonomous in terms of energy and communication networks, given the unreliability of these two resources. Yet, their size must be small enough to move in a constrained environment. For example, an autonomous vacuum cleaner must be quite narrow and small to pass under furniture. Solutions that allow decision-making autonomy must take into account the constraints related to energy autonomy, size and weight. Two solutions are emerging: model compression, which reduces the size of machine learning models, and frugal AI, which aims to create models that consume less energy. Euranova is positioning itself as a pioneer in this field, with research projects and several concrete applications underway.
Besides, applying AI/ML/Deep Learning to computer vision is an extremely trendy topic. There are hundreds of new papers a month on the topic; a two-year-old paper is already outdated. We constantly keep ourselves up to date with emerging topics.
Realising Your Vision
Ultimately, the success of a computer vision project depends on a thorough understanding of the specific needs and challenges of each application. By combining the right data collection, advanced algorithms and effective integration into embedded devices, it is possible to deploy innovative, value-creating solutions. Euranova stands out for its expertise and commitment to meeting these challenges, paving the way for new advances in the field of computer vision. We'll be happy to answer your questions, such as what the drones will be used for… OK, we are telling you this one because you read to the end: the drones give them the capacity to build their own datasets. We will use it to move our research projects further and provide our clients with data assets tailored to their goals.
If you're intrigued by the possibilities of computer vision and how it can elevate your business, don't hesitate to reach out to Guillaume and Quentin for more insights!